Data quality emerges as key barrier to GenAI adoption
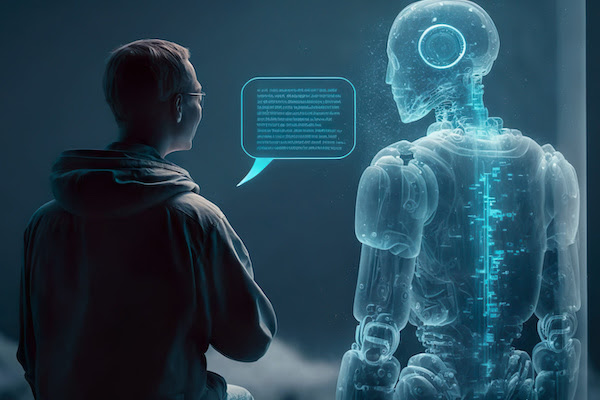
A recent report by Forrester reveals that data quality is now the primary factor limiting the adoption of generative artificial intelligence (GenAI) across industries. Since the emergence of GenAI, particularly with the release of ChatGPT in 2022, businesses have been eager to explore its potential for various use cases. However, the journey from experimentation to full-scale implementation has been hindered by challenges related to data quality.
Initially, GenAI adoption faced hurdles due to limited availability of ready-to-use applications and a lack of expertise among users. However, with advancements in technology, businesses now have access to a variety of large language models (LLMs) and user-friendly software platforms, making GenAI more accessible than ever before.
As we enter 2024, there is a noticeable shift towards launching GenAI into production solutions to cater to customer needs and enhance customer-facing roles. The ability of GenAI to summarise vast amounts of plain text and to generate creative content has captured the attention of the media, investors, and businesses alike.
Despite the progress in GenAI technology, data quality remains a significant challenge. The report highlights how the unpredictability of GenAI insights and the unprecedented speed, scale, and complexity at which it consumes data demand a new approach to data governance. Traditional methods of data management are no longer sufficient to ensure the accuracy and reliability of GenAI-generated insights.
To address the data-quality challenges associated with GenAI adoption, Forrester recommend businesses to adopt a holistic approach that combines technical expertise with domain knowledge.
Here at XBRL International, we are encouraging our global ecosystem of regulators, software vendors, the accounting profession and a wide variety of users, to combine AI technology with structured data sets. Our own experiments demonstrate positive results – with increased accuracy and improved outcomes – when using structured datasets with AI. We’ve only scratched the surface, but it is clear that the ability to interrogate huge numbers of structured reports prepared in XBRL — using plain language — is just around the corner. XBRL obliges the preparers of reports to provide structured data and sophisticated metadata. This combination is something of an AI goldmine and should greatly assist data quality.
Together, these technologies could unlock breakthrough analytic capabilities.
Read more here.