Structured data could unlock AI’s potential in finance
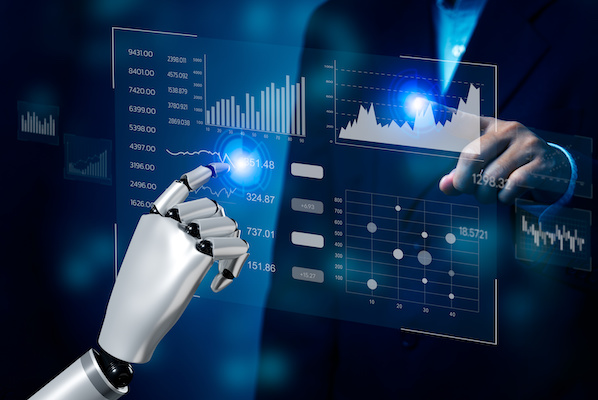
One item in particular over the holiday break caught our attention. Research by Patronus AI highlighted apparent challenges faced by large language models (LLMs), such as OpenAI’s GPT-4, in analysing financial data contained in US Securities and Exchange Commission (SEC) filings.
The study, focused on financial queries, found that even with access to extensive filings, the best-performing model, GPT-4-Turbo, achieved only a 79% accuracy rate.
But hang on… this is some of the best structured information on the planet! It’s all been tagged with Inline XBRL! What went wrong with their research? Oh. You guessed it. The researchers bypassed the structured information and used {Ed: Whimpers!} PDF versions of these financial statement filings contained on corporate websites.
So yesterday we did our own little experiment to understand if AI performance would improve when fed the structured data in Inline XBRL held at the SEC rather than PDFs. When XII’s team dived into the data, we found that AI systems like OpenAI’s GPT-4 demonstrate massively improved performance in answering financial queries when fed with xBRL-JSON converted from the SEC’s 10K Inline XBRL reports. Harnessing AI analysis of structured data offered vastly more accurate natural language query results in all of the areas that we looked at, including:
- Estimating the percentage of Cost of Goods Sold (COGS)
- Determining dividends paid to common shareholders
- Analysing customer concentration
- Assessing profit growth
- Evaluating capital expenditures
The researchers suggests (unsurprisingly) that large language models can struggle with unstructured data, often providing incorrect answers or even refusing to answer. Leveraging existing structured data, as in the case of SEC filings, is key to more reliable analysis by AI models.
The filings submitted to the SEC are already meticulously structured by companies, with XBRL data mandatorily embedded by corporate management in their disclosures. As extracting relevant financial information with AI is more effective when that information is structured, it would be wise to make use of this pre-structured data.
{Ed: Respectfully, starting analysis with PDF versions of 10K filings is ridiculous. It’s like printing out digital photographs of some fireworks and then cutting out individual letters with blunt scissors and gluing them onto the page to spell out the words “Happy New Year” on the paper. It’s 2024! Wake up and smell the structured data!}
Despite current limitations, the researchers believe in the long-term potential of language models like Chat-GPT to aid professionals in the finance industry. However, they stress the need for continuous improvement in AI models.
From our perspective, we would add the extremely obvious: improvements will be significantly accelerated by leveraging XBRL for enhanced accuracy and reliability in AI-based financial analysis. We just scratched the surface, over an hour or two… and we are sure our readers could do better. Start by converting the Inline XBRL into xBRL-JSON (most XBRL processors now let you do this extremely quickly), give the AI a few prompt hints about the structure and go from there. If we get time we’ll dig a little deeper and report back next week.
Read the article describing this research here.