Deep learning models in finance: trustworthiness and implications for regulation
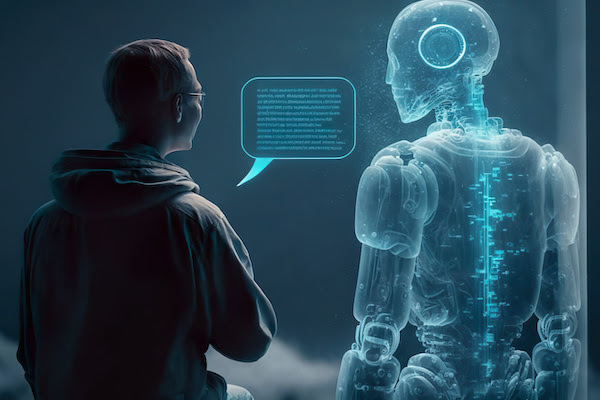
Deep learning models, often the bedrock of machine learning and artificial intelligence, are playing an increasingly large role in financial analysis. These models, while powerful, lack transparency – often known as ‘black boxes’ due to the opacity of their algorithms. A recent staff working paper by the Bank of England explores the implications of these kind of models on regulation and risk.
Results discovered by deep learning models are increasingly used for critical decisions. The study looks into the stability of predictions and explanations generated by different deep learning models, revealing interesting insights. Even when deep learning models differ only slightly in their settings, they produce similar predictions but different explanations. This divergence occurs even when variations are arbitrary. In contrast, traditional, transparent models deliver similar accuracies while maintaining consistent explanations and predictions.
This divergence in explanations and predictions among deep learning models, despite similar outcomes, poses important risk management questions. The findings suggest that while these models may be accurate, their opaqueness can hinder the understanding of how and why they arrive at specific conclusions.
This research underscores the importance of transparency and interpretability in the application of deep learning models within the financial sector – as well as the need for accessible, structured data to underpin analysis models.
Read more here.