Narrative disclosure analysis with GPT-4: unlocking new insights
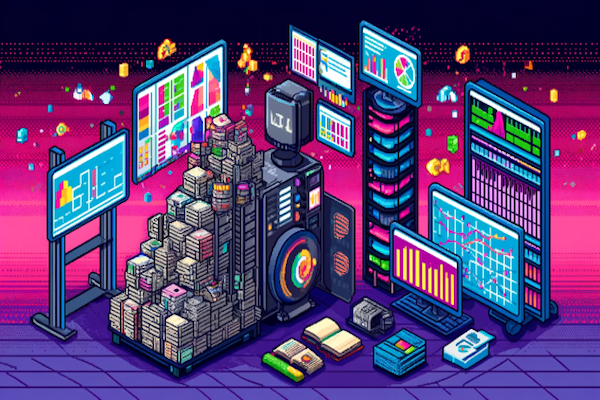
XBRL International’s own Revathy Ramanan dives into the potential of GPT-4 for analysing narrative disclosures in XBRL data this week. As machine learning and generative AI continue to advance, they present new opportunities for understanding complex business performance data. In particular, these new technologies can crack open relatively intractable data that is difficult to analyse conventionally, making it easier to access insights without deep technical knowledge.
In her last blog post Ramanan explored how GPT-4 can understand XBRL data and can be leveraged to do fundamental analysis using simple English queries. In this week’s post, she delves into what GPT-4 can do specifically with narrative data, or text blocks. Sustainability disclosures in particular are rich in narrative data and new analytic approaches are needed to effectively process this text-based information.
Unlike quantitative data, which includes numbers and statistics, narrative disclosures offer detailed explanation and context around a company’s performance, risks, and prospects. However, it is harder to compare and spot patterns in large amounts of narrative data.
GPT-4 can be used to analyse accounting policy texts from ESEF reports over consecutive years. The AI efficiently identified material changes in the text, demonstrating an ability to handle narrative comparisons. For going concern disclosures, GPT-4 calculated TF-IDF scores for 180 ESEF reports, highlighting the variations and helping to identify outliers for further investigation. This process, typically requiring statistical software and coding skills, was made accessible through simple queries in natural language.
Using data from India’s Business Responsibility and Sustainability Reporting (BRSR) mandate, GPT-4 was tasked with analysing the objectivity of risk mitigation disclosures. The AI classified the entries based on specific criteria, providing a clear distinction between subjective and objective statements. This kind of analysis could clearly be helpful to auditors and regulators alike.
These examples underscore how GPT-4 can democratise access to complex data analysis, enabling stakeholders to interact with data intuitively. The integration of Large Language Models (LLMs) with traditional data analysis opens new horizons for exploring data beyond predefined dashboards and static views. Clean, structured data is, however, crucial for accurate analysis, and the use of xBRL-JSON formatted reports enhances the reliability of insights generated by GPT-4.
Read the full blog to discover how narrative disclosure can be easily analysed in GPT-4 using natural language and without needing to know how to code.