Eurofiling: XBRL Data feeds Explainable AI Models
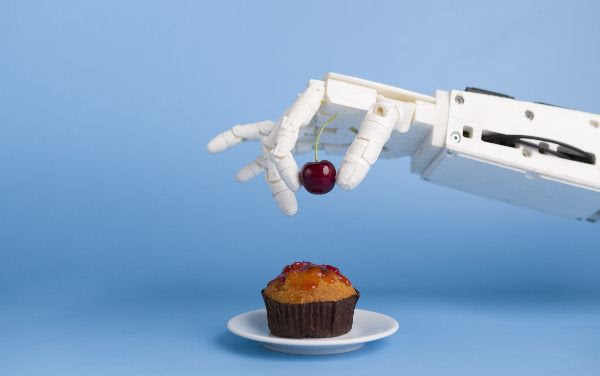
Amongst several fascinating presentations at the Eurofiling Innovation Day this week was an interesting demonstration on how XBRL reports can be used as the basis of explainable AI for bankruptcy prediction.
Dr. Maria Mora and Dr. Alicia Rodriguez, Fujitsu Laboratories of Europe, and Revathy Ramanan, XBRL International, discussed their study into the need for transparent bankruptcy prediction models based on accessible, quality data on financial performance. The study used 10+ years of XBRL annual and audit reports filed with the Spanish Securities Market Commission (CNMV) to build a machine learning (ML) model for predicting company distress.
The study used an explainable AI model to make the rationale behind the predictions clear. Explainable AI helps shed some much-needed light into the black box of AI algorithms by providing quantitative guides to how the various variables alter the final result (in this case the probability of risk, altered by changes in key financial concepts and liquidity, solvency and profitability ratios).
The presentation also highlighted how useful new xBRL-JSON and xBRL-CSV formats will be for leveraging the essential XBRL data needed to build effective machine learning models.
This was a great demonstration of how publicly available XBRL data can be used in AI and ML models not just to predict distress, but also to explain how the model detected the distress, increasing trust in the prediction and process.
More information on this presentation and other Eurofiling sessions can be accessed here.