Machine Learning Analysis of Big Data more effective for Credit Scoring
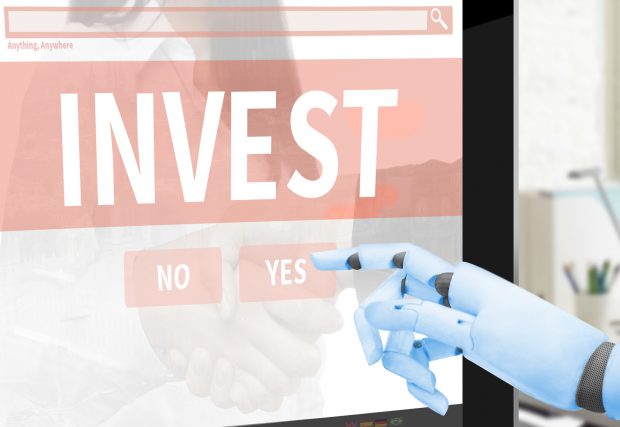
We’ve heard a lot about the potential of fintech to open up access to credit by using big data and alternative data sources to assess risk – but how do these techniques actually stack up when compared to traditional credit scoring?
A recent working paper from the Bank for International Settlements (BIS) looks at transaction level data from a leading Chinese fintech company to test the ability of different credit scoring models to predict losses and defaults.
Fintech credit is particularly well developed in China, with new credit scoring models making use of vast quantities of information from a wide range of data sources – including alternative data sources such as mobile data.
The BIS study looked at the predictive power of credit scoring models based on machine learning techniques compared with the traditional loss and default models typically used by banks. It found that the model based on machine learning and alternative data was better able to predict losses and defaults than traditional models during a time of economic shock – however, the comparative advantage declined for borrowers with a longer credit history.
Whichever model is used, more data meant more accurate predictions – and, for machine learning to be as effective as possible, vast quantities of structured data need to be made available. The potential for this technology to vastly increase market competition and access to finance is exciting – however, as many have previously cautioned, fintech must be properly regulated to mitigate economic risks, competition and privacy concerns.
Read the paper in full here.