Insights from Japan’s Digitally Tagged KAMs: 4 – Granular narrative tags are more useful
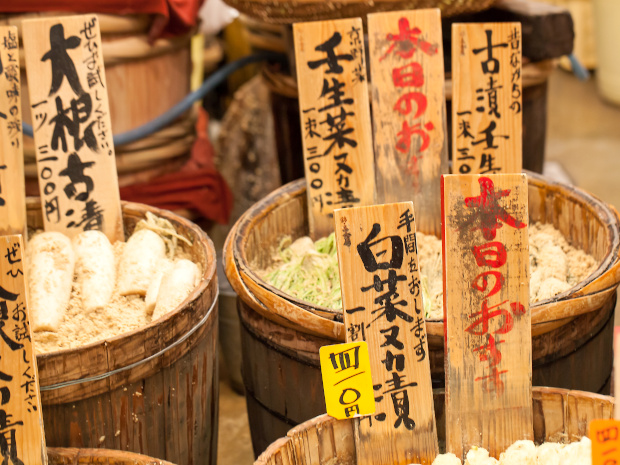
This is part of a short series on initial insights from our analysis of digital KAMs data being collected in Japan for the first time. For the first post and introduction, start here.
In the earlier posts in this series, we used specific digitally tagged segments of Key Audit Matters (KAMs) — called “Critical Audit Matters” or CAMs in some parts of the world — disclosures to derive insights about the risks and concerns identified in audit reports on consolidated financial statements from Japan. These included the ‘Short description’ and the ‘Audit response.’ In this post, we will explore what happens if we analyse the entire KAM text block, and compare this with the results from the “Short Description”. In other words, do more granular narrative tags help consumption?
When KAMs disclosures are prepared, the entire section is tagged as a single text block. Within that, each KAM – several may be identified, or just one or two – is also tagged with specific data points, namely the short description of the KAM, KAM content and reasons, auditor response, and note reference.
The following graphic compares KAM keywords automatically extracted from the specific ‘Short description’ tag with those extracted from the full ‘Key audit matters text block’ tag, in both cases using consolidated reports filed by banks.
It is clear at a glance that the keywords and keyphrases extracted from the specific ‘Short description’ tag provide relatively focused insight on the topics of interest to auditors, cutting through the noise. Also, note that the keywords are relatively intuitive and logical in terms of the concerns they reflect, given that the data in this case comes from the banking sector.
On the other hand, the keywords from the entire text block are more generic and less accurate, with relatively few of the same keywords (shown in red) also appearing in this list. Here, to get more meaningful results, the model extracting data from the text block would need to be adjusted to remove common terms (known as stop words). This fine-tuning is typically iterative, making it time-consuming, and often involves judgement on what to leave out, leading to biases.
Using the more granular narrative tag, we have quickly been able to generate useful insights on real-world risks of concern to auditors. Our comparison suggests that granular narrative tagging can be much more informative and more straightforward to analyse – and confirms that analytics on large chunks of text can be challenging.
This is the final post in the series. We hope that this initial analysis has begun to demonstrate some of the insights made possible by digital tagging of KAMs – and perhaps even encouraged you to explore further analytical possibilities.
We offer these as key take-aways from the series:
- KAMs help us understand risks identified by auditors and provide perspectives on areas of concern across and within industry sectors.
- Structured, machine-readable data makes analysis hugely easier.
- Digital tagging of narrative KAMS disclosures using XBRL opens up opportunities to analyse this data and derive insights that could be useful to investors and other users alike.
- For textual data such as this, a range of techniques such as sentiment analysis, clustering, keyword extraction, and cosine similarity can draw out patterns and provide insights across large datasets.
- Granular tagging provides distinctive breakdowns that are analytically more useful than block tagging.
Please note that the data was originally in Japanese and was translated using Google Translate, so it may well contain translation errors. It is also early days for this new tagging requirement, so we can expect some data-quality issues; we understand that the Japanese Institute of Certified Public Accountants is currently working on some relevant guidance. We thank XBRL Japan for sharing the data, enabling us to set the ball rolling with this initial analysis.